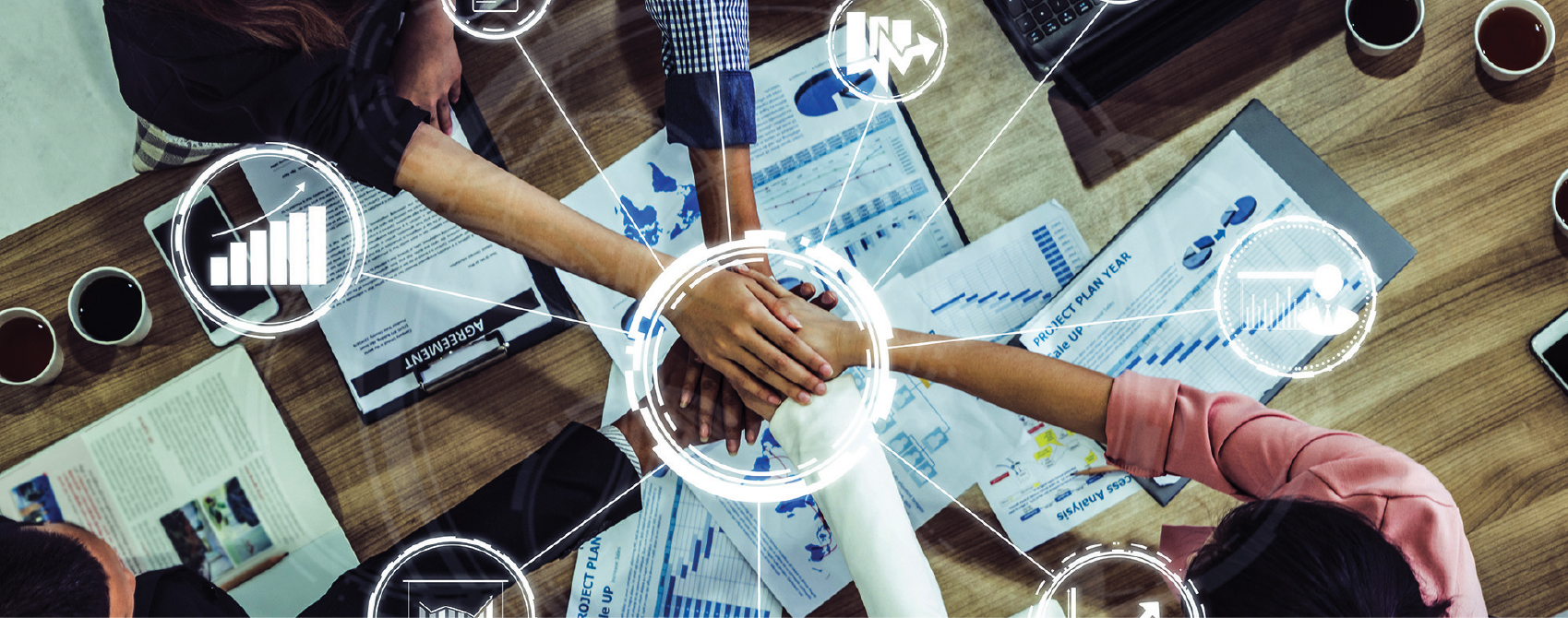
Project Management TRAINING
Click For All Business Training Courses "Rick was a fantastic instructor, great class keeping us engaged and the material interesting. He made everyone feel comfortable, I thought he was a great instructor"
May 2025 | AZ-140T00 Student
VIEW COMMENTSAfter class is over, re-watch
the recording 24/7 with Replay